Applying IDW interpolation and explore the modifications based on the measured temperature from the weather stations in Italy, 2006.
IDW Interpolation
IDW interpolation, which stands for Inverse Distance Weighting interpolation, is a widely used method in geostatistics for estimating values at unmeasured locations based on the values observed at nearby locations. It is particularly useful in various applications, including weather forecasting. The exercise was made to apply IDW interpolation based on the measure temperature from the weather stations in Italy, 2006.
The basic idea behind IDW interpolation is that the value at an unmeasured location is estimated by assigning weights to the measured values at nearby locations, with the weights inversely proportional to their distances from the target location. In other words, closer measured locations have a greater influence on the estimation compared to farther locations.
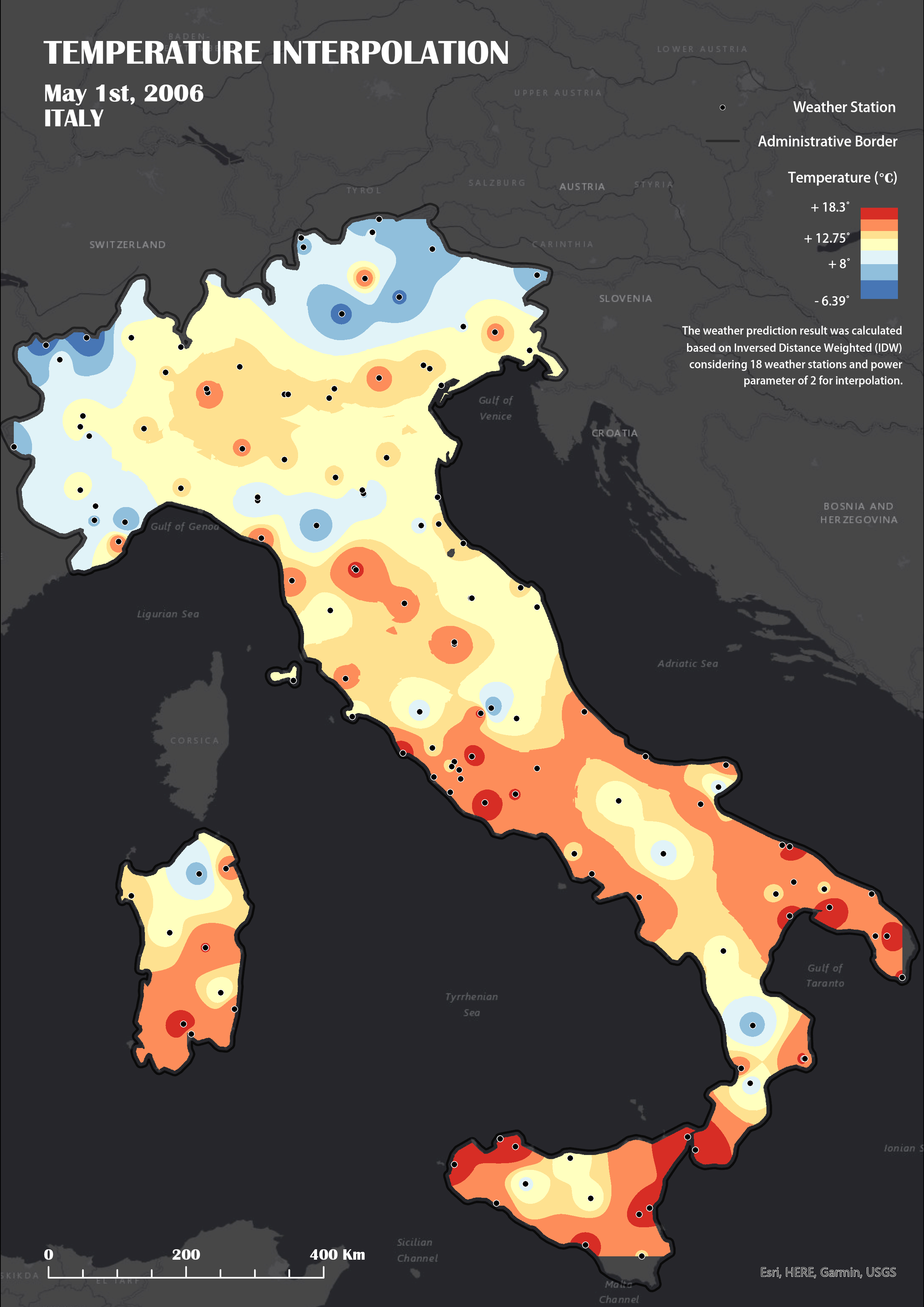
In addition, the output could be influenced by Power factor.The weight attributed to each known observation is controlled by a power parameter, often referred to as p. In fact, if p is small, neighboring and distant observations exercise a strong influence on unsampled locations. As a consequence, the prediction might be heavily smoothed because it does not solely consider local processes. On the other hand, if p is set too high, only the very local phenomena will be accounted for and the interpolated map might present a jagged appearance. The map below shows the influence of higher P values on the interpolation result.
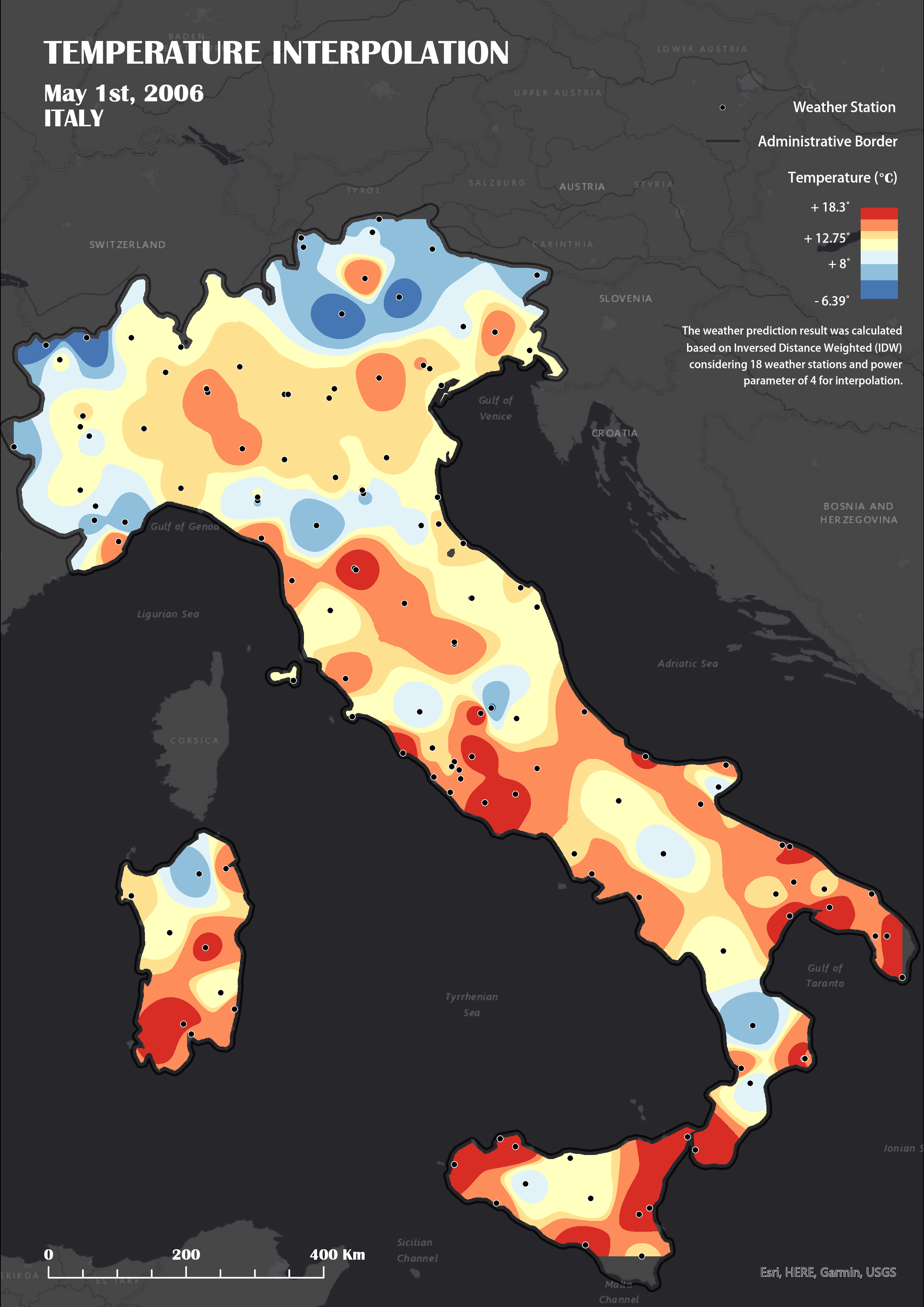
It's worth noting that while IDW interpolation is a simple and widely used method, it has certain limitations. It assumes that the measured values have a spatial correlation, which may not always be the case. Additionally, IDW interpolation does not consider other factors that may affect the spatial variability of weather variables, such as topography or land cover. Therefore, more advanced interpolation techniques, such as kriging or Gaussian processes, are often employed in conjunction with IDW to improve the accuracy of weather forecasts.